Any views or opinions presented in this article are solely those of the author and do not necessarily represent those of the company. AHP accepts no liability for the content of this article, or for the consequences of any actions taken on the basis of the information provided unless that information is subsequently confirmed in writing.
Introduction
Health care spending across the country generates billions of claim records annually. Claim records originate as a form of invoice for health care providers to bill for services rendered. Providers submit the claims to the liable payer (typically a health plan, government agency or the patient). The health plans adjudicate the claims, pay the providers, and then store the electronic claim records in data warehouses. Regulations require that health plans keep claim records for several years, typically ranging from five to 10+ years depending on the state. Many health plans choose to keep their records indefinitely, and expand their storage capabilities as needed. The IT infrastructure require to hold and maintain electronic records is one of the biggest administrative expenses for health plans. Since health plans incur significant expense to store their claim records, is there a way that health plans can use the data to help run their business more effectively or help in achieving the triple aim (i.e., patient satisfaction, population health and cost containment)?
Actuaries have traditionally used health plan claim records to build actuarial cost models. Actuarial cost models are a staple tool used by health actuaries to assist with financial forecasting, which is then used for financial planning and pricing benefit coverage. Using historical claims data, the actuary constructs cost utilization reports by splitting the data into homogeneous service categories, and then summarizing metrics such as claim frequency, unit costs, provider discounts, per-member per-month (PMPM) cost, actuarial value (AV), medical loss ratio (MLR) and risk score. The reports can be further partitioned by line of business, market segment, time frame, or any other desired attribute of the covered population. The information in the reports is then incorporated into the Actuarial Cost Model to estimate future health care costs and serve the traditional actuarial functions of pricing and forecasting. However, while these functions are vital to the financial stability of health plans, they do not influence health care costs or the way health care is delivered.
Actuarial cost models can be powerful tools to help influence health plan decisions. Actuaries can dig deep into the claims data to explore solutions to myriad medical economic problems related to total cost of care. Even though the claim records are intended mainly for invoice purposes, they contain valuable clinical and financial information that can be used to help guide health plan leadership in making smart business decisions. The first step to getting at this information is to create actuarial cost models that help health plan leadership understand the total cost of care. The second step is to create more detailed models that highlight the areas that most influence the total cost and will help guide health plan leadership to make actionable business decisions to lower the cost of care.
In the remainder of the article, I explore six example applications of actuarial cost models. In each example health care claim records are a critical element of the model, and in several of the examples it is imperative that actuaries work closely with clinicians to either help build the model or help interpret the model results.
- Provider specialty cost model. Supports the analysis of provider efficiency, benchmarking and network adequacy.
- Primary care physician (PCP) cost model. Supports the analysis of attribution, PCP performance and value-based contracting.
- Clinical cost model. Supports the analysis of treatment plan costs, and the evaluation of care management programs.
- Enterprise risk management (ERM) cost model. Supports the analysis of quantifying the financial risk associated with risk-bearing contracts.
- Site-of-service cost model. Highlights the opportunity to shift procedures to a lower-cost setting.
- Inpatient diagnosis-related group (DRG) cost model. Highlights the opportunity to reduce inpatient lengths of stay.
Provider Specialty Cost Model: Efficiency And Network Review
Claims data can be used to create actuarial cost models that track the cost of care for every provider within a network. This provider specialty cost model will produce separate cost profiles for each individual provider (or provider group), and then compare to other providers within the same specialty category. The specialty categories are homogeneous groupings based either on the provider taxonomy code or Centers for Medicare & Medicaid Services (CMS) specialty code that is typically found in the claims data. This modeling may be limited to physician specialties, but it can also be applied to health care facilities (e.g., hospitals, clinics, ambulatory surgical centers) as well as non-physician practitioner (e.g., dentists, physical therapists, nurse practitioners). The provider cost profile reports will give actuaries important information to help them better understand the provider landscape, such as:
- Number of providers within each specialty
- Total volume each provider receives
- Types of services each provider performs
- Comparison of utilization metrics (visit frequency, unit cost, and mix of procedures) for each provider within each specialty
Actuaries can then use that information to identify cost differences between providers. They will need to consult with a clinician to most effectively interpret the meaning of the model results (e.g., understanding various treatment plans for common ailments), and, together, draw conclusions on how to best make actionable decisions from the results. Such actions may include:
- Creating provider benchmarks that can be used to help guide contract designs and negotiations
- Developing treatment plan “playbooks” that represent cost-efficient practices
- Identifying a lack (or surplus) of access of certain specialties within an area, which can guide leadership in how to address an underlying deficiency (or surplus) in the network. If the network needs to be expanded, the model can be used to track the performance of new providers; if the network needs to be contracted, the model results can help with the decision-making
PCP Cost Model: Value-Based Contracting
The PCP cost model has many of the same attributes as the provider specialty cost model, but has a couple of important distinct features. First, the model is limited to just PCPs. Second, each member is attributed to a unique PCP for a defined time frame. Third, all the member’s claim experience for that time frame is credited toward the attributed PCP. Attributions are typically defined on either a monthly or annual basis. There will be some members who do not get attributed because they either have no claims in the study period or have claims but just not any PCP claims. It is very important to note that the PCP is credited for all the claim experience of his attributed members. This includes all specialist visits, prescription drugs, emergency room visits, hospital admissions and out-of-network utilization. Certain costs may be excluded from the attribution. The excluded costs are usually limited to services that the PCP is not able to influence. Typical examples are transplants, mental health, skilled nursing and out-of-area claims. Like the provider specialty cost model, monitoring reports can be created, benchmarks can be established, and comparisons between PCPs can be made.
The results of this model will help health plan leadership make informed decisions regarding their network of PCPs. Decisions can range from whether to expand or reducethe number of PCPs within a region, choosing PCPs to be part of a tiered network, or how to approach negotiations for risk-based contracts and value-based payment (VBP) programs. PCPs have the most influence over their patients’ health care costs, and because of this, are the best targets for VBP. The results of the PCP cost model give health plan leadership the information needed to help design and negotiate contracts that will reward the quality of care instead of rewarding the quantity of care. The actuary and clinician together can review the details of PCP attribution costs and develop budget targets for the PCP to receive bonuses and withhold refunds. A well-designed VBP arrangement should incent PCPs to manage and direct the care of their patients in a cost-efficient manner while maintaining high-quality care and patient satisfaction. A clinician’s expertise is vital to ensure any goals related to cost efficiency do not sacrifice the quality of care delivered to patients. The PCP cost model will help health plan leadership with the financial aspects of designing a quality VBP arrangement, including budgeting and monitoring emerging results.
Clinical Cost Model: Intervention Program Evaluation And Targeting
The clinical cost model is constructed by mapping health plan members into homogeneous clinical condition categories based on the diagnosis codes that are present on each member’s claim experience. Since a member may have various diagnoses, the categories can either be hierarchical, where the member is placed into the category corresponding to the most serious condition, or the categories can be subdivided based on a member’s various co-morbidities. This model helps to illustrate the number of members within each clinical category, the total cost of treatment, the mix of services, and the incremental cost of co-morbidities. The actuary can use this model to help evaluate the financial impact of offering various medical intervention programs to guide health plan leadership in narrowing down their choice of programs to offer.
Actuaries would use the model results as a starting point in their evaluation, but may need to go back to the claims data and adjust the model depending on the type of members the program is intended to target. For example, an intervention program may be designed to target members who had a heart attack within the past six months but do not also have diabetes (perhaps because diabetics are already eligible for an alternate program). The clinical cost model would have a category for members with heart disease, but would not have a category specific to the target population. The actuary must go back to claims data to find members who have a principal diagnosis code for acute myocardial infarction within the specified time frame, and make sure to exclude any member who also has diagnosis codes related to diabetes. Now that the member list has been narrowed to the targeted population, the actuary can develop an actuarial cost model to financially evaluate the program. Next the actuary will summarize the historical claim experience for the target members and develop claim reduction estimates by applying assumptions such as member take-up rate, claim trend, and adjustments for clinical intervention (including regression to the mean).
In addition to helping with the evaluation of disease management programs, this model will also help to monitor the acuity of a population over time, the progression of disease, and the cost difference between various treatment plans. The actuary and clinician together can review the model results to develop treatment plans based on the observed historical cost and outcomes of the various treatment plans for a specific condition.
ERM Cost Model: Risk Simulation
Past claim experience is a good predictor of future claim experience, but there is variance from year to year that can be difficult to foresee. Unpredictable future costs associated with catastrophic events (e.g., epidemics), benefit changes (e.g., new mandates and high-cost drugs) and new populations (e.g., previously uninsured) may destabilize claim trends and lead to significant financial losses. To help quantify a potential range of financial outcomes, claims data may be used to create an ERM cost model that stochastically simulates annual claim cost for a block of business. First, the actuary needs to construct a claim probability distribution from the claims data, then use Monte Carlo simulation to randomly sample the distribution thousands or even millions of times, with each sample representing a single member’s annual claim cost. If a block of business has 100,000 members, the model would sample the distribution 100,000 times independently to simulate the total claim costs for the block.
Since this is a stochastic model, the results will vary every time the model is run. To create a range of plausible results, the sampling process should be repeated several times. For example, 100 independent runs (each run simulating the claims for 100,000 members) will give the actuary a good feel for the range of risk for a block of business. The result of each run would represent a different percentile of the distribution of total claim cost risk (e.g., the scenario with the 10th highest aggregate claims would represent the 90 percent percentile of risk).
Different claim distributions should be used for blocks of business with distinct characteristics and utilization patterns. For example, Medicare claims should not be used to simulate risk for a commercial population, and vice versa. The actuary may also introduce additional random variables into the model to recognize that the assumed claim distribution may change from year to year. For example, a random variable can be used to scale either the mean or dispersion of the distribution. The model may also run simulations for multiple blocks of business together, or multiple years in succession. Many health plans like to produce three- to five-year forecasts, so running a simulation for all lines of business for three to five years in succession will provide a better understanding of the aggregated risk than running separate simulations for each line of business and separately for each year.
The output of the model is a distribution of potential financial outcomes and the statistical likelihood of each outcome. The results will help health plan leadership become more aware of the aggregated risk of their business and provide critical information for them to decide their appetite for risk. Plan leadership may use the results to:
- Decide to either increase or decrease their exposure in certain markets
- Decide if reinsurance is appropriate, at what attachment level, individual or aggregate, or both
- Create financial forecasts
- Fulfill risk reporting requirements, such as Own Risk and Solvency Assessment (ORSA)
Site-Of-Service Cost Model: Most Efficient Place Of Service
Claims data can be used to create site-of-service cost models that help to identify which services have the most opportunity to shift to a lower-cost place of service, and then model the financial impact of the shift. First the actuary will need to identify the applicable procedural codes for a common set of services (e.g., a common set of surgeries, cancer treatment, preventive services). Next the actuary will need to identify all claims containing the relevant set of procedural codes, and then summarize the data into an actuarial cost model that shows cost and utilization metrics for those visits by the place of service. Most acute medical services are performed either in a doctor’s office, hospital inpatient setting, hospital outpatient setting, or at a non-hospital facility (e.g., ambulatory surgical center, clinic). The cost for a given procedure will typically be the lowest if it is performed in an office and the highest if performed in the hospital inpatient setting, with the hospital outpatient and non-hospital facility costs falling in between the two extremes. The model will show the number of procedures performed in the various settings and the cost difference between each. The actuary can then model the potential cost reduction by shifting a percentage of the procedures to a lower-cost setting.
The model results will help guide health plan leadership to implement medical policies that require that certain procedures are to be performed in the lowest-cost setting unless there is a medical necessity for a higher-cost setting (e.g., procedure carries a higher-than-normal risk due to patient co-morbidities or frailty). For example, the model may show that certain treatments in Region A are expensive because they are mostly performed in the outpatient setting, whereas the same treatments are cheap in Region B because they are mostly performed in offices. A clinician will then assess why Region A is using more outpatient treatment and decide if it is feasible to shift more treatment to the office setting. Combining the results of the provider specialty cost model with the results of the site-of-service cost model, health plan leadership may see a need to expand their network or the need for a new facility in the region. For example, if Region A has a high frequency of low-level emergency room visits compared to Region B, the reason may be because there is an urgent care facility in Region B but not in Region A, or because Region B has PCPs with weekend office hours and Region A does not. Depending on the situation, health plan leadership has the information needed to make decisions.
DRG Cost Model: Inpatient Length Of Stay Reduction
Hospital inpatient stays are typically reimbursed through a bundled payment referred to as a DRG (Diagnostic-Related Group) payment. A DRG payment is designed to reimburse the hospital for a patient’s entire stay at the hospital regardless of the length of stay (LOS) or the amount of resources consumed. The DRG only covers hospital expenses, and does not cover the costs associated with physicians that bill for services separately (e.g., surgeon expenses). The two most common DRG systems used in the United States today are the Medicare Severity (MS-DRG) and All Payer Refined (APR-DRG). Both systems determine a bundled payment based on a combination of diagnoses and procedure codes. The combination of these codes will reflect the case complexity and the required course of treatment, which then correlates to an expected consumption of hospital resources and length of treatment.
An actuarial cost model can be created from claims data that compares the average length of stay (ALOS) by DRG code between all the hospitals within a network. The actuary can then work with a clinician to create benchmark ALOS for each DRG, and model the claim cost reduction by assuming the ALOS for each hospital will converge toward the benchmark (assuming cost per day remains the same). For example, if the actual LOS for a certain DRG is 6.0 days at Hospital A, and the benchmark LOS for that DRG is 4.0 days, then a 25 percent marginal improvement would reduce the LOS by 0.5 day. If Hospital A had 500 admissions for that DRG averaging $5,000 per day, then there would be a cost savings potential of $1.25 million. The patient will consume fewer hospital resources with a shorter LOS (reducing costs for the hospital), but the health plan will not realize any immediate savings since DRGs’ are bundled payments. However, if the hospitals are successful in reducing the ALOS, health plan leadership may be able to use the model results to negotiate a better DRG weight and share the savings with the hospitals. Aside from the financial implication, reducing ALOS will get patients home sooner, and will free up hospital resources for other patients.
Conclusion
The above examples are just a starting point for all the valuable analysis that can be done with health care claims data. The actuarial models that can be created, combined with a clinical perspective, will provide health plan leadership with the analytics needed to monitor their business and make the decisions necessary to transform health care into a high-quality and cost-efficient delivery system. I cannot stress enough how important it is for actuaries to team up with clinicians. Actuaries are experts at modeling data, but clinicians are experts at delivering care. The combined technical expertise of actuaries and medical expertise of physicians are critical to addressing issues related to the triple aim (i.e., patient satisfaction, population health and cost containment).
About the Author
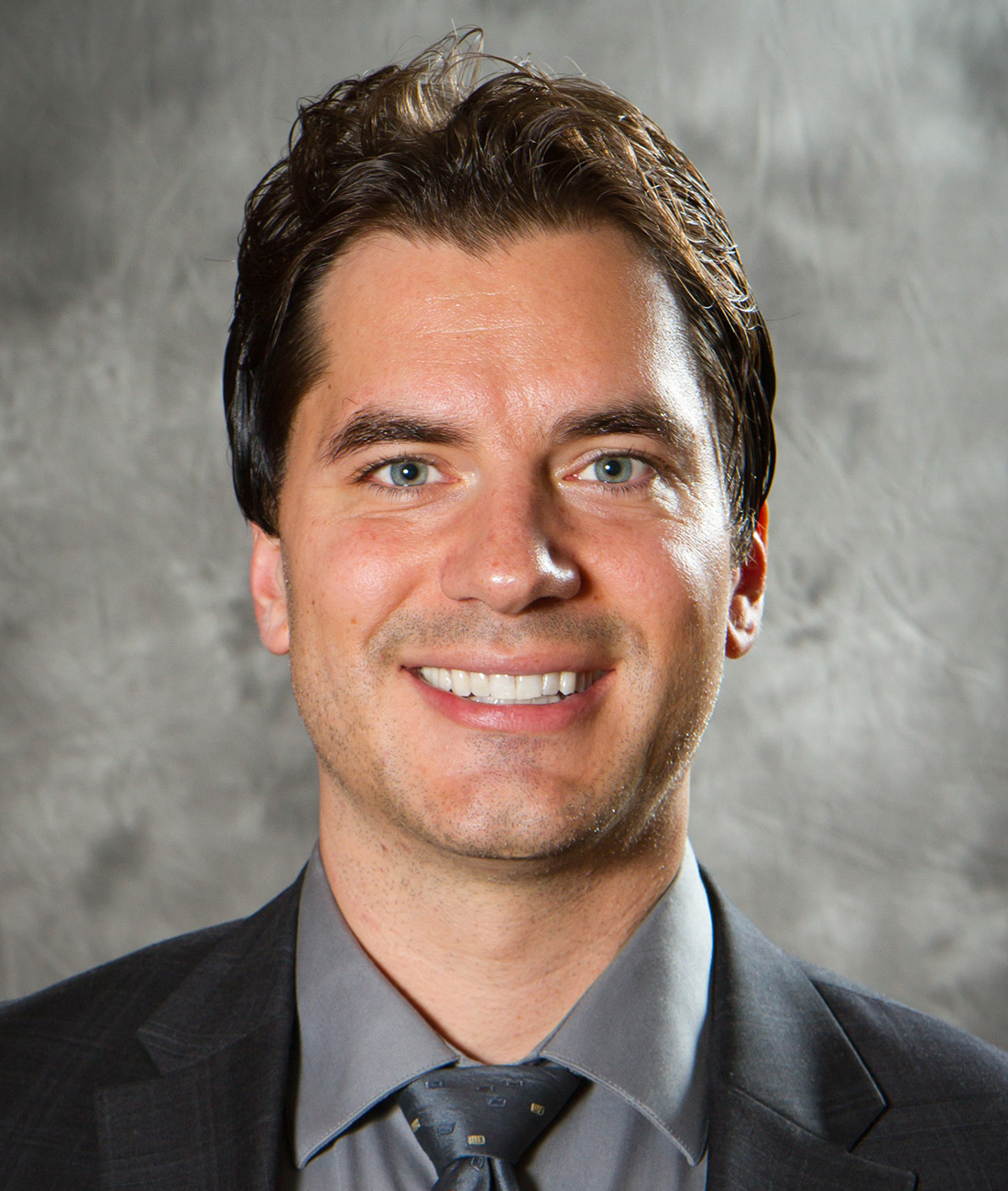
William Bednar, FSA, MAAA is a Consulting Actuary at Axene Health Partners, LLC and is based in AHP’s Murrieta, CA office.