Summary
Determining an ROI for any care program or intervention can be challenging, but the challenge was multiplied for a children’s hospital implementing a new care program for the sickest children enrolled in Medicaid managed care. The program targeted a relatively small number of patients, and ongoing sustainability relied on the participation of the managed Medicaid insurers covering the population. In effect, the hospital wanted to convince the insurers that the program was financially sustainable. At the same time, the hospital and insurers wanted to ensure that key quality and patient satisfaction criteria were met. Working with the hospital, affiliated academic researchers, and actuaries from the participating insurers, we developed a comprehensive evaluation and measurement strategy, along with supporting calculations that demonstrated that the program indeed generated financial value over a 3-year period while meeting quality and patient satisfaction goals.
The purpose of this material is to describe briefly the measurement strategy and share the general methodology by which the financial results were determined. Some residual challenges and opportunities are discussed. The author would be happy to discuss more details with respect to the methodology and challenges with any interested readers.
Measurement Strategy
The first step was the development of a measurement strategy that would take into account the concerns of the hospital as well as the insurers. There was a clear need to demonstrate the financial performance of the program. We also wanted to measure key utilization statistics as “corroborating evidence” supporting the financial performance evaluation. Finally, we identified key quality and patient/caregiver satisfaction criteria to be measured for the program.
The result was a multi-pronged measurement platform:
- Financial Outcomes (the primary focus of this material)
- Utilization Reduction – e.g., reductions in emergency department utilization; all-cause readmission rate; and average length of stay
- Process Measures & Specific Interventions – e.g., metrics regarding program enrollment; care manager contact; plans for seizure patients; and asthmatics with 2 or more asthma office visits
- Patient/Caregiver Experience & Outcomes – e.g., results from FECC[1]; PedsQL[2]
- Quality Metrics – e.g., % patients with at least one well-child visit per year; prevalence of ambulatory sensitive inpatient stays
The intent was to capture these metrics on a quarterly basis. As noted in the introduction to this material, measurement was carried out over a 3-year period. While the overall measurement platform stayed relatively static, the individual metrics evolved as the program progressed.
Assessing Financial Outcomes
Over the course of this assignment, we explored different avenues for evaluating the financial impact, if any, of the hospital’s intervention program. The final methodology for determining financial outcomes was built around a control-group approach, and was deemed acceptable by both the hospital and the insurers.
Basic Methodology
The fundamental point of analysis was the comparison of growth (i.e., trend) in PMPM costs in the intervention group versus the control group. Underlying data – detailed claims and eligibility – was provided by the Medicaid insurers for their covered populations. Key steps in the analysis were:
- Identify the intervention group. The hospital team identified eligible members based on key characteristics (e.g., number of ER and IP visits in the past year) and tracked the members who enrolled in the program. The list of members and months enrolled was provided to the analytics team from the hospital team at the close of each quarter.
- Select the control group. The intervention program was focused on two specific counties. To generate a control group, a third county was identified with similar geographic and access to care characteristics. Academic researchers affiliated with the hospital then selected control group members based on criteria we identified with the hospital team.
- Calculate PMPM growth over the 3-year time period for each group versus the original baseline period, with adjustments for:
- Claims completion – to ensure we captured expected claims that had been incurred but not yet paid
- Annual trend – to reflect typical growth of Medicaid costs in the state
- Catastrophic claimants – to remove the impact of one-time “hits” to the experience over time (e.g., a claimant with more than $50,000 in claims in a single month was removed from eligibility and claims experience for that month)
- Risk – to reflect changes in the apparent risk over time, as well as to account for differences in risk between the intervention group and the control group
- Compare the PMPM growth to determine whether the intervention program resulted in a differential financial impact.
When we completed this exercise, we found that the intervention group demonstrated a 19.6% reduction in PMPM costs, versus a 0.6% reduction for the control group. This result thus suggests that the program saved 19% over the 3-year period.
In our calculation, we recognized that certain factors were highly leveraged – in particular, the impact of catastrophic claimants and risk differences (3.c. and 3.d. above) were large drivers of the results in our calculations. When we removed the influence of those factors, we arrived at a more conservative estimate of savings – approximately 5%. Thus, our final assessment was that the program saved between 5% and 19% of health care costs for the target population.
Residual Challenges and Opportunities
While the financial evaluation points to favorable results for the hospital’s program, there remain several issues for anyone trying to replicate these results.
Small size of target group and control group
This hospital’s program targeted the sickest children enrolled in Medicaid in two counties. Starting with a baseline population of about 3,000 children, between 400 and 700 were enrolled in the intervention program in any given month. This raised the question of credibility for this size of group. In an effort to address this concern, we used multiple years’ experience in our calculations. We also looked to corroborating evidence – reductions in IP and ER utilization, for example – to support the apparent results. Regardless, critical reviewers will appropriately point to the small group size as a potential problem for generating credible financial results.
Applicability of standard risk score methodologies for a pediatric population
For risk adjustment, we used the PRISM (Pediatric Risk of Mortality) score as reported by the state to each of the insurers for each member. When measured against this population, we found that the square of the PRISM score (PRISM2) fit best with the experience.
Many analytics teams will look to commonly available risk assessment/adjustment models to provide a risk score for this kind of calculation. Such models are usually built around primarily adult populations. It is absolutely critical to evaluate how well the particular model measures pediatric risk.
Attribution of apparent savings to different programs
The insurers recognized the value of the intervention, but also pointed to programs that each insurer themselves had implemented to help manage risk. More than one insurer suggested that the results obtained by the hospital’s program might in some part be due to earlier care management efforts at the insurer level.
We attempted to document pre-existing programs, but insurers varied in their ability to report to us which members had been enrolled in their programs. This issue was somewhat mitigated by the fact that we evaluated the program over a period of three years. However, the question remains as to whether the financial results reflect other programs in addition to the hospital’s intervention.
Integration of behavioral health
In an ideal situation, behavioral health data would be integrated into the medical and pharmacy claims, to allow for a more holistic view of health care costs. In this hospital’s state, behavioral health was carved out completely from the Medicaid insurers into a separate program, and thus we were not able to get that experience.
General availability of control group data
Perhaps the most common challenge in pursuing this methodology is finding an appropriate source for a control group. Access to normative detailed databases can be helpful, but in this situation, there were no resources available to supply a reasonable control group for a very sick Medicaid pediatric population. Working with the insurers and hospital, we were able to identify a separate county from which the control group could be generated.
In Closing
This particular assignment focused on a very specific situation – a pediatric hospital program for Medicaid child enrollees. However, the general methodology can be applied for a wide range of interventions and populations. The challenges described above may exist for many professionals seeking to analyze the financial impact of a particular program or intervention.
[1]Family Experiences with Care Coordination measure set, supported by the AHRQ-CMS Pediatric Quality Measures Program
[2]Pediatric Quality of Life Inventory, measuring health-related quality of life
About the Author
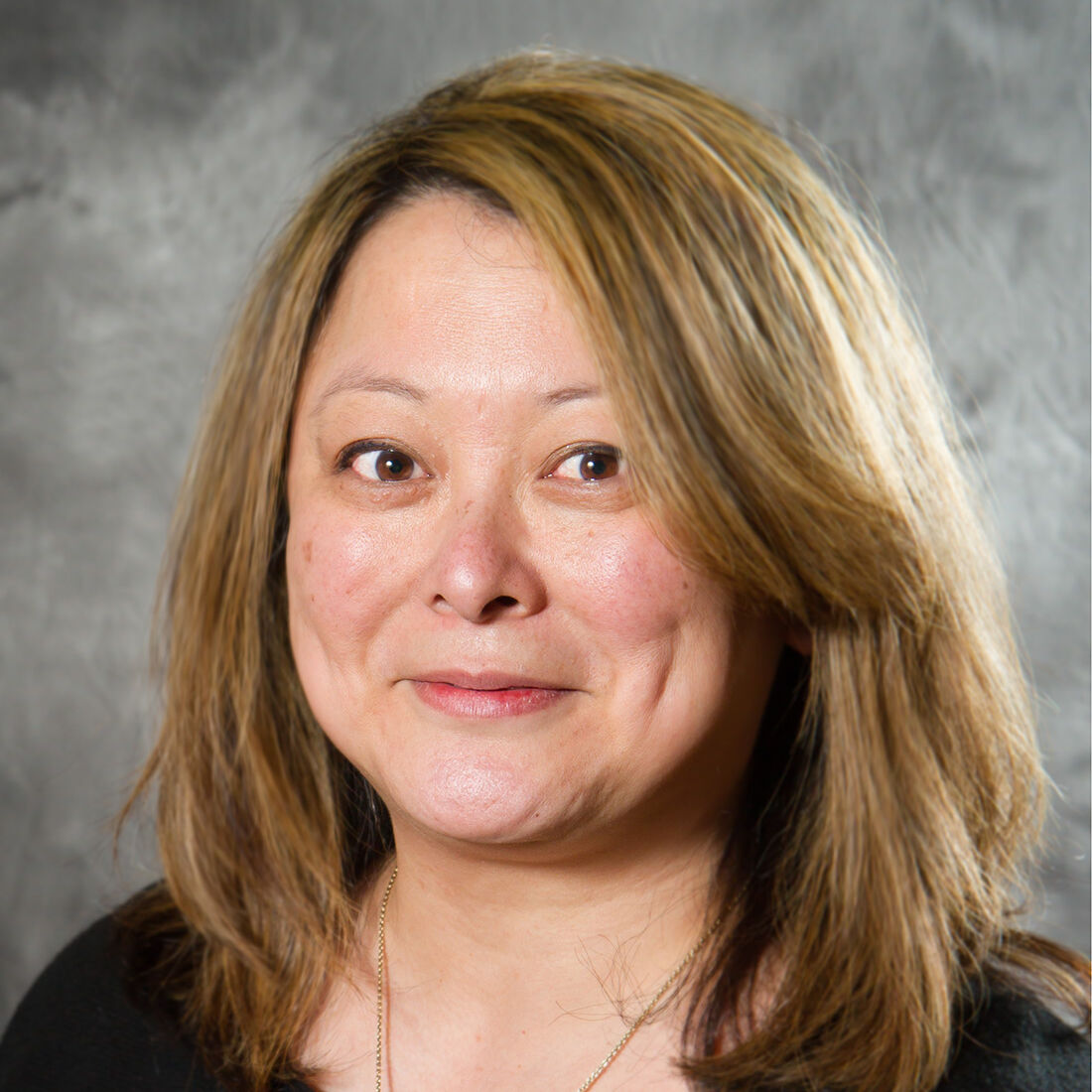
Guest Post – Elaine Corrough, FSA, FCA, MAAA
Any views or opinions presented in this article are solely those of the author and do not necessarily represent those of the company. AHP accepts no liability for the content of this article, or for the consequences of any actions taken on the basis of the information provided unless that information is subsequently confirmed in writing.