As states have begun to relax social distancing measures, some feel a sense of optimism. People here in California are going to beaches and restaurants (although those activities generally still have some social distancing requirements). While many individuals are already looking forward to once again enjoying their pre-lockdown life, COVID-19 modelers are starting to sound alarm bells once again. Death projections are ticking back up from previous lows. The IHME model’s death projections have doubled[1], and widely circulated preliminary results from a Johns Hopkins model suggest that the daily death count may rise substantially above the current plateau of about 1,800 deaths per day. The infamous 3,000 deaths per day prediction from the Johns Hopkins model is now known to be based on an incomplete set of scenarios[2], but the directionality of the change seems intuitive given the lifting of some protective orders.
COVID-19 updates have dominated the global news cycle for months now. But even though consuming pandemic news feels like you are drinking from a firehose, there is still so much that is unknown about the disease. For this and other reasons[3], most COVID-related models still have a wide range of estimates for healthcare costs[4], hospitalizations5, deaths[5], and more. Below, we will discuss how variations in some still-unknown model parameters can have dramatic effects on death predictions.
For example, we know that average daily reported deaths in the United States for the past two to three weeks have been about 1,800[6]. (As noted in other articles[7], reported deaths are likely an undercount of the true death total, but we will calibrate to reported totals for the sake of the illustrations here). We used the AHP COVID-19 Planning Resource Model (The AHP CPR Model) to generate scenarios that would result in about 1,800 deaths per day as of May 1, 2020. We started with three hypothetical scenarios as described below.
There is an important caveat before we dive into the illustrations: the nationwide estimates below were calculated assuming the infection growth rate and overall mortality rates are consistent across the United States. We assume this for simplicity as our intent here is to illustrate the potential impact of assumption changes, not to hypothesize actual future death counts. The spread of the virus depends on hyper-regional factors such as population density and adherence to social distancing measures, while the infection fatality rate depends heavily on age and underlying health conditions. All of these factors vary considerably across the United States. Nationwide projections are at their best as the sum of individual pieces, given local variations in these critical variables.
Now that we have the big caveat out of the way, we can look at some hypotheticals. We’ll consider two classes of assumptions:
- Factors that we have little control over
- Factors that we have some control over
Factors that we have little control over include things like the infection fatality rate (IFR). Scientists developing therapeutics have some control over this, doctors administering life-saving care have some control over this, but most of us regular people do not.
Factors that we have some control over include things like the future growth rate of new cases. A community’s adherence to protective measures can have a dramatic impact on the growth rate of new cases.
Variables in each of these two classes are generally unknown, though data is constantly improving. As we’ll show below, given the explosive nature of exponential growth models, changes to any variable can have dramatic effects on projections. So it’s important to be aware of how any model is treating each variable, and whether the assumptions are reasonable. We simply make the distinction between the two classes of assumptions because, as we will see, changes to factors that we have control over can have just as great an effect on death projections as changes to factors that we do not have control over. And in a time of apparent chaos, it can be comforting to realize there are still things in our control.
Assumption Class 1: Factors that we have little control over
In all scenarios below, we initialize the model on March 1, 2020 with the starting cases shown in Figure 1 and a case doubling rate of 3 days. We also assume for all scenarios that the doubling time has graded to 30 days by May 1, 2020[8]. For simplicity, we then hold the doubling time at 30 days thereafter. In other words: we assume that the doubling time (or, equivalently, the infection growth rate or R0[9]) in place as of early May will not get better or worse as the year progresses. Finally, all scenarios assume 14 days from infection to death. In fact, we only vary three parameters in order to generate our dramatically different scenarios, as shown in Figure 1:
Figure 1: Sample Model Parameters
The reader will notice that the starting number of cases varies quite a bit. The number of known cases as of our March 1, 2020 start date is 40, per The COVID Tracking Project. This is about 10-20% of the numbers shown above. However, due to limited testing availing on March 1, 2020, the number of known cases is almost certainly understated. A reported-to-actual case ratio of 10% has been hypothesized by some researchers, although estimates of the true ratio vary widely[10].
Figure 2 shows the impact on daily deaths on the 1st of each month for the remainder of 2020, again assuming no change to the case doubling rate from the current levels. Note the infectious period is labeled T_inf, and we have removed the y-axis as our focus here is on relativities rather than counts.
Figure 2: Impact of Infection Fatality Rate and Infectious Period on Daily Death Totals
Scenario 2 has half of the infection fatality rate of Scenario 1, yet the daily deaths in this scenario range from 30% to 100% of Scenario 1. This is in part due to the lagging nature of deaths. In the first scenario, we start with about half the cases of the second scenario. As such, both peak infections (not shown) and peak deaths are pushed out farther. Deaths in Scenario 2 start declining as those in Scenario 1 hit their peak. This shift in the peak exacerbates the difference in daily death counts.
Comparing Scenario 2 to Scenario 3 shows the impact of a change in the length of time that an infected individual can spread the virus to others. The CDC reports that the period of infectiousness is not yet known, but the incubation period is likely 14 days or less[11]. Lower lengths for the infectious period result in fewer cases and thus fewer deaths, as shown in Figure 2.
As we discussed earlier, most of us can’t do anything about the assumptions detailed in Figure 1. However, differences in modelers’ opinions about those assumptions will affect those modelers’ death projections, so it’s important to be aware of the values of these assumptions when reviewing model output.
Assumption Class 2: Factors that we have some control over
The most important of these factors is the infection growth rate (or case doubling time, or R0). We can affect this parameter via mask-wearing, hand-washing, and a number of other actions recommended by public health officials.
We will take Scenario 3 from Figure 1 as a baseline scenario and add some optimistic and pessimistic variations. Note we will use the doubling rate and R0 interchangeably in these scenario descriptions as they are a function of one another.
- Scenario 3: Assume doubling rate holds at 30 days throughout 2020 (associated R0 of 1.2).
- Scenario 4: Assume case doubling rate drops to 20 days on May 15. Assume doubling rate toggles between 20 and 30 days every month thereafter as restrictions are lifted and re-imposed.
- Scenario 5: Starting on May 15, assume R0 is 0.9 (meaning infections will decline). Assume R0 toggles between 0.9 and 1.1 each month thereafter as restrictions are lifted and re-imposed.
- Scenario 6: Starting on May 15, assume R0 = 1, so each infected person infects one other person only. Assume this persists throughout 2020.
Figure 3: Impact of Infection Growth Rate on Daily Death Totals
Note that regardless of the scenario, the daily deaths on June 1, 2020 are higher than those on May 1, 2020. This is because deaths lag infections, and we assume an increasing rate of infections until May 15 in all the above scenarios.
Figure 3 demonstrates that future death totals will be greatly impacted by whether we continue to curb the growth rate of new infections.
There are many other factors that affect death rates: resource availability, testing capacity to the extent it helps us better understand the infection growth rate and perform effective contract tracing, and more. Investigating the impact of these factors might be done in a later article.
What do we do now?
We do not have control over the duration of the infectious period or many of the other inherent characteristics of the virus. But we do have control over our actions. To the extent that we can slow the spread of the virus by acting carefully, we can collectively reduce potential fatalities throughout 2020.
And when we’re taking a break from washing our hands to review current COVID model outlooks, we should keep in mind: model output is a function of model input. A good model maker is transparent about their methods and assumptions, and a good model user is aware of those methods and assumptions. Be a good model user, because the assumptions are critical. In fact, our lives depend on them.
Endnotes
[4] https://axenehp.com/the-cost-of-covid-19/
[6] Data pulled from The COVID Tracking Project at covidtracking.com on 5/6/2020.
[7] https://axenehp.com/data-data-everywhere-drop-drink/
[8] As of May 5, doubling time was about 26 days for the U.S. overall, and trending upward.
[9] We are using terminology from the primer here: https://plus.maths.org/content/maths-minute-r0-and-herd-immunity. We will use R0 instead of R because we want an apples-to-apples comparison of our assumed infection growth rate at different points in the timeline, independent of the percent of the population still susceptible to the disease.
About the Author
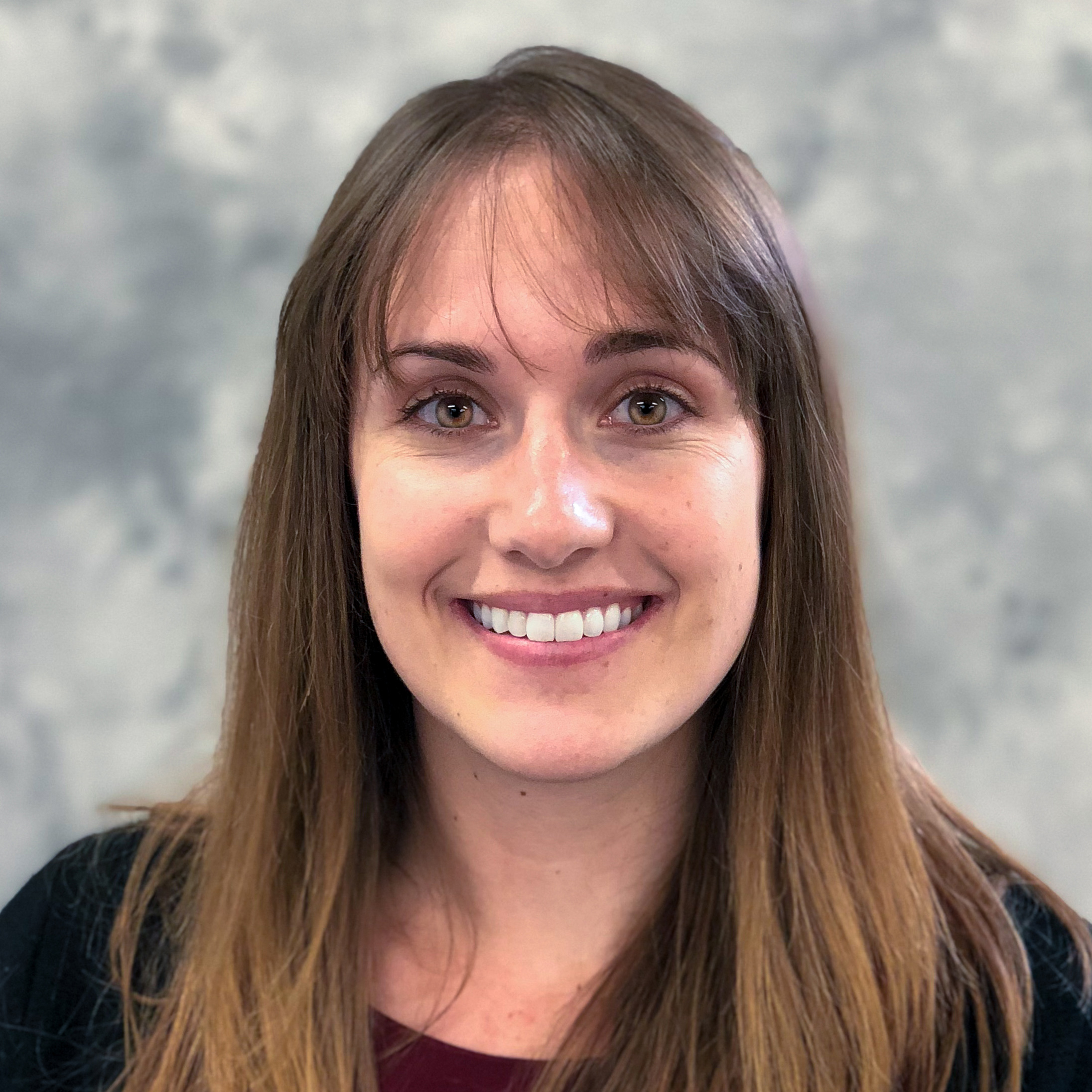
Any views or opinions presented in this article are solely those of the author and do not necessarily represent those of the company. AHP accepts no liability for the content of this article, or for the consequences of any actions taken on the basis of the information provided unless that information is subsequently confirmed in writing.